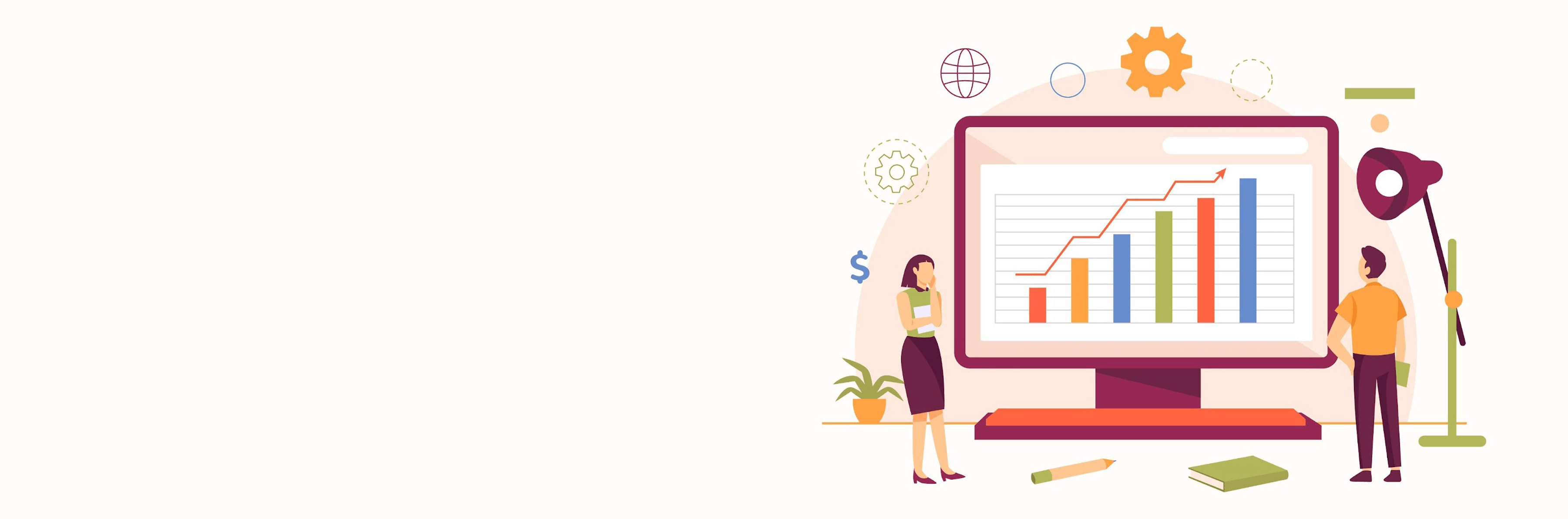
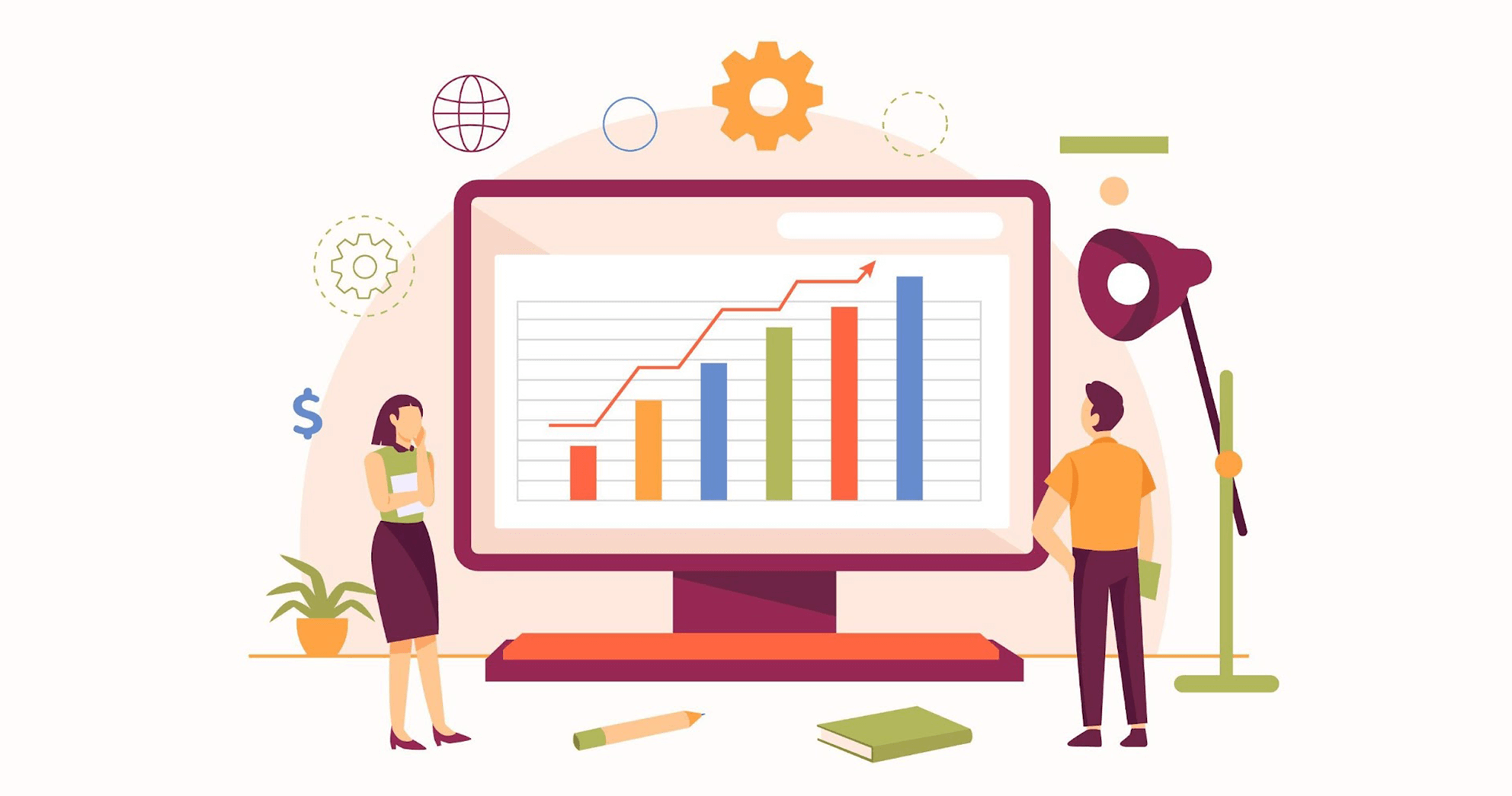
March 2, 2023
This is blog 2 of 3 in our FAIR model series. The limitations of FAIR’s data collection process are discussed in part 1 of this blog series.
Building a lego design and quantifying cyber risk have essential characteristics in common. To construct a lego design, you start by collecting the necessary pieces and arranging these pieces by shape, color, structure and function. From there, you assemble these pieces to create a boat, race car, or that killer spaceship. In the same way, to perform cyber risk quantification (CRQ), you collect data and feed it into a statistical model to put the “pieces together” and produce a quantified risk calculation in monetary terms like dollars.
FAIR (Factor Analysis of Information Risk) is an industry-recognized CRQ methodology. Using a top-down approach, an analyst uses FAIR to create and analyze risk scenarios from your environment one at a time to determine their potential loss exposure to your organization. The FAIR model provides a framework to decompose these risk scenarios by different factors and acts as a guide to explain the relationships of risks within an organization. Connecting these risk scenarios to the FAIR model requires collecting large volumes of data and input from scenario-related experts or consultants. You can utilize this data to surface assumptions about the risk scenario and feed the data into a Monte Carlo simulation model to estimate the financial impact of risk in dollars.
The FAIR risk model helps organizations identify and define the very high-level building blocks that make up risk, but its methodology alone does not support how to set assumptions properly. This leads to challenges around implementing FAIR in practice, making it difficult for organizations to accurately calculate their financial loss exposure. This is due to three reasons:
Setting assumptions is a critical component of the FAIR risk calculation process. Once you define the risk scenario you want to quantify, analysts can use the FAIR model, shown in the flowchart below, to scope assumptions about the risk scenario and specify the minimum, maximum, and most likely loss exposure values.
FAIR’s risk assessment is performed in four steps, each outlined in the diagram below. The first three steps involve manually collecting data related to the scoped risk scenario. The last step analyzes the collected data using Monte Carlo simulations to estimate the financial impact in dollars. Setting assumptions for each scenario occurs after data is collected and before it is fed into a Monte Carlo simulation.
With FAIR, the assumption setting is left to the user, and often the user needs insights to make these assumptions. Even if the user has collected extensive data about the probable risk scenario or consulted with experts, certain risk factors within the FAIR model are difficult to determine and estimate, such as Resistance Strength and Threat Capability. This can lead to users coming up with wildly varied and undefendable answers. With a great deal of human intervention and input involved in this process, assumptions tend to be subjective, leading to questionable outputs produced by the model. Moreover, the sheer number of assumptions required to calculate risk with FAIR reduces the credibility and defensibility of the analysis.
Operationalizing the FAIR model to determine how loss might unfold for a possible risk scenario is manual and resource-intensive. Organizations need to define a set of considered risk scenarios, use the flowchart to assess each risk factor, collect appropriate information, make assumptions about the scenario, and then evaluate their estimated loss exposure. In some situations, organizations may also hire an assessor or a consultant to help them define their risk scenarios and facilitate conversations about their loss exposure.
Organizations looking to speed up this process can adopt an analysis platform on top of the FAIR model. These platforms systematically guide analysts through a FAIR analysis with data points collected from their organization and other external sources. These platforms also run this data through a FAIR model so users can estimate their risk exposure in financial terms. While this sounds great in theory, deploying software on top of FAIR may require extra budgeting and employee training to sustain. Moreover, maintaining the FAIR model in real-time is nearly impossible – risk scenarios need to be constantly re-analyzed based on changes to the organization or technology environment. Data also needs to be collected as new threats emerge, and new assumptions need to be made as risk scenarios evolve.
An organization’s cyber risk evolves daily, with new threats emerging faster than ever. Continuously monitoring your attack surface is the only way to understand your organization’s risks. This is why it’s essential to have a CRQ model that accounts for threats in your network as soon as they emerge.
One of the significant drawbacks to the FAIR model is that it is fairly static – requiring quite a bit of manual analysis and expertise to make updates and adjustments or to add new dimensions to any threat analysis. On its own, the FAIR model does not automatically update as your attack surface evolves. This is largely due to FAIR’s top-down approach to CRQ which utilizes scenarios and high-level subjective assumptions to estimate risk in monetary terms. With a top-down approach, risk modeling is simplistic and rudimentary at best and incapable of automatically adjusting threat scenarios based on the current state of your network’s assets, their roles, and criticality and mitigating controls.
FAIR’s top-down approach differs from a bottoms-up asset-level CRQ model that typically utilizes machine learning to calculate risk. With machine learning, a CRQ model can quickly analyze millions of events and identify many threats – like malware exploiting zero-day vulnerabilities or Log4j instances. Machine learning technologies also automate the process of discovering new assets, categorizing and determining the business impact of those assets, and associated vulnerabilities and their threats, detecting controls and estimating their efficacy, and continually calculating breach likelihood. This type of approach also allows for a granular CRQ model that automatically adjusts threat scenarios analyzed based on your environment’s latest asset activity, their respective roles and business criticality, and any mitigating controls deployed on the assets. This model can also automatically account for vulnerabilities and threats based on near real-time instances of vulnerabilities found within the environment and correlated threat intel. Here is where the FAIR approach falls short, making it challenging for users to accurately incorporate information about specific threats and exploits in real-time into their risk calculation. As a result, FAIR’s risk calculation does not capture anywhere close to the true nature of an organization’s risk environment.
Balbix is a platform that enables a bottoms-up, asset-level risk model for near-real-time cyber risk quantification. The Balbix risk model is maximally automated and leverages data you already have in your network to continuously calculate your cyber risk in monetary terms. With Balbix, you can also slice and dice risk by business unit, by site, by the owner and trace to the underlying issues driving risk, while gaining actionable insights for risk reduction. FAIR does not provide either of these capabilities effectively.
While many other CRQ vendors utilize FAIR, or a close variant, Balbix’s risk model takes an entirely different approach to cyber risk quantification because:
With Balbix, your cyber risk is continuously quantified in dollars by analyzing real-time asset-level data from your existing tools, including vulnerabilities, threats, exposure, security controls, and business criticality. Balbix then uses specialized AI and automation to analyze, normalize and correlate your data to continuously discover and monitor your devices, apps, and users; and calculate your overall cyber risk posture based on breach likelihood and impact. Balbix’s risk score updates in real-time from the state of your enterprise’s software and systems and as new vulnerabilities are discovered, providing you with an accurate and concrete understanding of your specific risk landscape.
Balbix provides enterprise-wide visibility of your assets and determines their importance to your organization. All of this is done automatically without the need to reassess risk manually. Balbix’s risk model calculates the likelihood of a data breach and the impact of a breach to your business – on a per-asset and per-vulnerability basis. The risk equation below shows that breach risk considers five critical factors. The first four factors calculate breach likelihood: vulnerability severity, threat level, asset exposure and security controls. The fifth factor, business criticality, is used to calculate breach impact and incorporates four impact cost categories: detection and escalation costs, notification costs, post-breach response costs, and lost business costs. Breach impact automatically establishes the relative business criticality of each asset by examining each asset’s type, roles, how it interacts with the rest of the network and other key attributes.
With the power of AI, the Balbix risk model automatically ingests real and objective data from your environment and threat data from external threat intelligence feeds. Using this data, Balbix automatically calculates cyber risk based on the expected financial loss resulting from a cyber attack or data breach by considering the likelihood and impact of a breach. As data is continuously ingested from your environment, your risk calculation is updated to reflect your current breach risk in monetary terms. Balbix also works to continuously evolve and improve its risk model to identify threats faster and with greater accuracy to reflect every component of your risk environment in its risk calculation.